Big Data Challenges To Data Warehouse
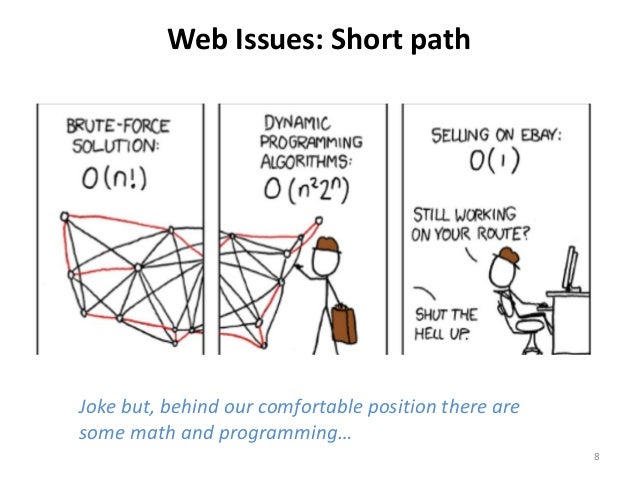
However, one of the reasons big data is so underutilized is because big data and big data technologies also present many challenges. One survey found that 55% of big data projects are never completed. This finding was repeated in a second survey, that found the majority of on-premises big data projects aren’t successful.
Jan 19, 2016For decades, the enterprise data warehouse (EDW) has been the aspirational analytic system for just about every organization. It has taken many forms throughout the enterprise, but all share the same core concepts of integration/consolidation of data from disparate sources, governing that data to provide reliability and trust, and enabling reporting and analytics.
A successful EDW implementation can drastically reduce IT staff bottlenecks and resource requirements, while empowering and streamlining data access for both technical and nontechnical users.The last few years, however, have been very disruptive to the data management landscape. What we refer to as the “big data” era has introduced new technologies and techniques that provide alternatives to the traditional EDW approach, and in many cases, exceeding its capabilities.
Many claim we are now in a post-EDW era and the concept itself is legacy. We position the EDW as a sound concept, however, one that needs to evolve. Challenges With the Traditional EDWThe EDW implementation itself can be a fairly difficult task with a high risk of failure. Generally accepted survey data puts the failure rate somewhere around 70%.
And of the 30% deemed nonfailures, a great number never achieve ROI or successful user acceptance. To a great extent this has been caused by legacy interpretations of EDW design and traditional waterfall SDLC.
Big Data Challenges Today
It’s safe to say more modern, agile techniques for design and implementation prove more successful and offer a higher ROI. These techniques allow EDW implementations to grow organically and be malleable as the underlying data and business requirements change.The fundamental issue is that traditional EDW does not solve all problems.
In many organizations, the EDW has been seen as the only solution for all data analytics problems. Data consumers have been conditioned to believe that if they want analytics support for problems, their only choice is to integrate data and business processes into the EDW program. At times, this has been a “cart before the horse” situation when extreme amounts of effort have been put into modeling new use cases into a rigid and governed system before the true requirements and value of the data are known.In other cases, the underlying design and technology of the EDW does not fit the problem. Semi-structured data analysis, real-time streaming analytics, network analysis, search, and discovery are ill-served by the traditional EDW backed by relational database technology.For more articles on the state of big data, your guide to the enterprise and technology issues IT professionals are being asked to cope with in 2016 as business or organizational leadership increasingly defines strategies that leverage the 'big data' phenomenon.Use cases such as these have become more common in the era of big data. In the “old days,” most data came from rigid, premise-based systems backed by relational database technology.
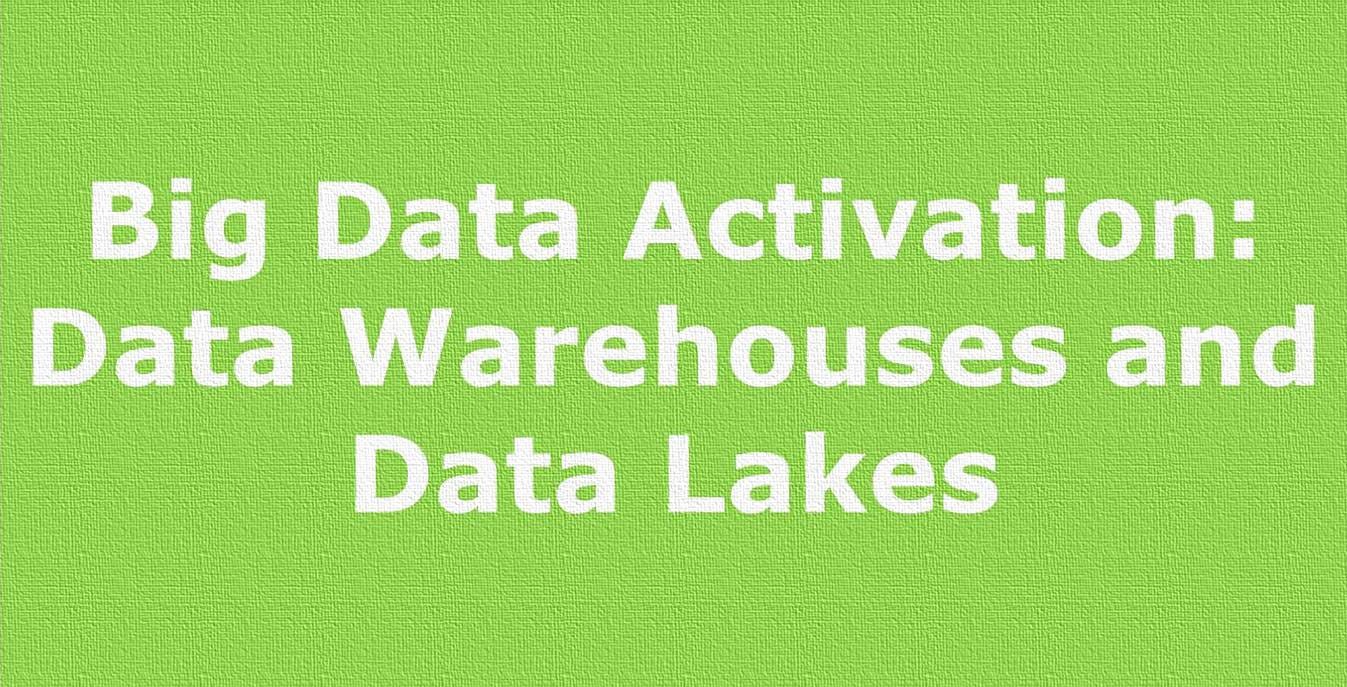
Although these systems still exist, many have moved to the cloud as SaaS models. In addition, many no longer run on relational platforms, and our method of interaction with them is often via API with JSON and XML responses. Additionally, there are new data sources, such as social, sensor and machine data, logs, and even video and audio. Not only are they producing data at overwhelming rates and with inherent mismatch to the relational model, there is often no internal ownership of the data, making it difficult to govern and conform to a rigid structure. The Big Data RevolutionIn response, there has been an amazing disruption in the tools and techniques used to store and process data. This innovation was born in large tech companies such as Twitter and Facebook and continues to rapidly evolve as all organizations realize similar challenges with their own data. Today, the excitement of the big data era is not just about having lots of data.
What’s truly interesting is that organizations with all data sizes now each approach data problems in different and tailored ways. It’s no longer a one-size-fits-all shoehorn into traditional systems. Organizations now objectively design and build systems based on business and data requirements, not on preconceived design approaches.
Why data lakes are an important piece of the overall big data strategy. By Prashant Tyagi (left) and Haluk DemirkanIn today’s complex business world, many organizations have noticed that the data they own and how they use it can make them different than others to innovate, to compete better and to stay in business 1. That’s why organizations try to collect and process as much data as possible, transform it into meaningful information with data-driven discoveries, and deliver it to the user in the right format for smarter decision-making 2. Big data analytics has become a key element of the business decision process over the last decade. With the right analytics, data can be turned into actionable intelligence that can be used to help make businesses maximize revenue, improve operations and mitigate risks.Big data is the term for a collection of data sets so large and complex that it becomes difficult to process using hands-on database management tools or traditional data processing applications. The challenges include capture, curation, storage, search, sharing, transfer, analysis, visualization and many other things.
According to Gartner, 60 percent of companies say they don’t have the skills to make the best use of their data.Photo Courtesy of 123rf.com Bruce RolffIDC predicts revenue from the sales of big data and analytics applications, tools and services will increase more than 50 percent, from nearly $122 billion in 2015 to more than $187 billion in 2019 3. Even though 73 percent of companies intend to increase spending on analytics and making data discovery a more significant part of their architecture, 60 percent feel they don’t have the skills to make the best use of their data 4. Given an abundance of knowledge and experience, combined with successful data and analytics-enabled decision support systems, big data initiatives come with high expectations, and many of them are doomed to fail.Research predicts that half of all big data projects will fail to deliver against their expectations 5. When Gartner asked what the biggest big data challenges were, the responses suggest that while all the companies plan to move ahead with big data projects, they still don’t have a good idea as to what they’re doing and why 6. The second major concern is not establishing data governance and management 7 (see Table 1). Trapp, a professor at the Foisie Business School at Worcester Polytechnic Institute (WPI), received a $320,000 National Science Foundation (NSF) grant to develop a computational tool to help humanitarian aid organizations significantly improve refugees’ chances of successfully resettling and integrating into a new country.
Built upon ongoing work with an international team of computer scientists and economists, the tool integrates machine learning and optimization algorithms, along with complex computation of data, to match refugees to communities where they will find appropriate resources, including employment opportunities.